USING NEURAL NETWORKS IN MODELING THE FINANCIAL RESULTS OF BUSINESS PROCESSES
Анотація
Introduction. The attributes of neural networks are embodied in a study of the effectiveness of business processes, which is based on integrated coefficients of international monitoring with a range of quantitative parameters. Simulated situational precedents will allow to assume multivariate solutions in real time.
The purpose of the work is to use of neural network technologies in modeling financial results of business processes with integrated international monitoring indices and domestic statistics.
Results. The obtained sections of the response surface of the resulting indicator and pairs of independent variables for a neural network of type RBF 3–7–1 are characterized. An algorithm is proposed for applying the methodology for assessing the functioning of a business using neural network technologies.
Conclusions.
1. According to the results of theoretical generalizations, the understanding of the main purpose of the business operation has been improved. A feature of the proposed interpretation is the narrowing of the functional component of business processes to the resulting feature in real time.
2. Low indicators of network readiness, level of ICT development, global competitiveness of the domestic economy and business profitability have been established.
3. For the simulated situations, the results obtained allowed to bring the convergence of the resulting indicator of relatively independent factors, that is, the response of domestic business to the intensification of digitalization, increasing the competitiveness of the economy and the development of information and communication technologies.
4. The paper proposes an algorithm for applying the methodology for assessing the functioning of a business using neural network technologies.
Ключові слова
Повний текст:
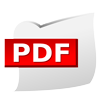
Посилання
Tudoroiu, N., Churu, C., & Grigire, M. (2008). Neural Networks architectures for modeling and simulation of the economy system dynamics. IEEE Trans Neural Netw. (Vol. 19). (Iss. 9). Sept. 2008, 1549–1563.
Maditinos, D. & Chatzoglou, P. (2004). The use of neural networks in forecasting. Review of Economic Sciences, 6, TEI of Epirus, 161–176.
Yu, L., Huang W., Lai K.K. (2007). Neural networks in finance and economics forecasting. International Journal of Information Technology and Decision Making, 1, 113–140. (Vol. 6).
Falat, L. & Pancikova, L. (2015). Quantitative modelling in economics with advanced artificial neural networks. Procedia Economics and Finance. 194–201. (Vol. 34).
Tello-Leal, E., Roa, J., Rubiolo, M., & Ramirez, U. (2018). Predicting activities in business processes with LSTM recurrent neural networks. 26-28 November, Santa Fe, Argentina, 13.
Iqbal, M. F., Zahid, M., Habib, D., & John, L. K. (2019). Efficient prediction of network traffic for real-time applications. Journal of Computer Networks and Communications. (Vol. 2019). Article ID 4067135, 11 p.
Kurochkina, I.P., Kalinin, I.I., Mamatova, L.A., Shuvalova, E.B. (2017). Metod neyronnyih setey v modelirovanii finansovyih pokazateley kompanii [The method of neural networks in modeling the financial performance of the company]. Statistika i economika – Statistics and Economics. 5, 33–41. (Vol. 14) [in Russian].
Guryanova, L. S., Gvozdytskyi, V. S., Dymchenko, O. V., Rudachenko, O. A. (2018). Models of forecasting in the mechanism of early informing and prevention of financial crises in corporate systems. Financial and credit activity: problems of theory and practice. 26, 303–312. (Vol. 1).
Andryushchenko, K. A., Rudik, V. K., Ryabchenko, O. P., Kachinska, M. O., Marinenko, N. Yu., Shergina, L. A., Kovtun, V. P., Teplyuk, M. A., Zhemba, A. I., Kuchai, O. V. (2019). Protsesy upravlinnia informatsiinoiu infrastrukturoiu tsyfrovoho pidpryiemstva v mezhakh kontseptsii “Industriia 4.0”. [Process management information infrastructure of the digital company in the inter-concept of “Industry 4.0”]. Shidno-Yevropeiskyi Zhurnal peredovyh tehnolohii – Eastern-European Journal of Enterprise Technologies. 3(97), 303–312. (Vol. 1) [in Ukrainian].
Maita, A. R. C., Martins, L. C., Paz, C. R. L., & Peres, S. M. (2015). Process mining through artificial neural networks and support vector machines: A systematic literature review. Business Process Management Journal, 6, 1391–1415. (Vol. 21).
Francescomarino, C. Di, Ghidini, C., Maggi, F. M., & Milani, F. (2018). Predictive process monitoring methods: which one suits me best? 16th International Conference, BPM 2018, Sydney, NSW, Australia, September 9–14, 462–479.
Ofitsiinyi sait Derzhavnoi sluzhby statystyky Ukrainy [Official site of the State Statistics Service of Ukraine]. (2018). Available at: http://www.ukrstat.gov.ua/.
The networked readiness index. Reports 2010–2011, 2012, 2013, 2014, 2015, 2016 of World Economic Forum. Available at: http://www3.weforum.org.
Measuring the information society reports 2014, 2015, 2016, 2017. The official web-site of ITU Telecom. Available at: https://www.itu.int/.
Osovsky, S. (2002). Neyronnyie seti dlya obrabotki informatsii [Neural networks for information processing] (Trans. I. D. Rudinsky). Moscow: Financy i Statistika [in Russian].
Kruglov, V. V. & Borisov, V. V. (2002). Iskusstvennyie neyronnyie seti. Teoriya i praktika [Artificial neural networks. Theory and practice]. (2nd ed.). Moscow: Goryachaya liniya. Telecom [in Russian].
Kasuk S. T. (2014). Analiz i prognozirovanie sportivnyih dannyih v neyronnyih setyah [Analysis and forecasting of sports data in neural networks]. Chelyabinsk: Uralskaya Akademiya [in Russian].
DOI: https://doi.org/10.35774/sf2019.03.108
Посилання
- Поки немає зовнішніх посилань.
West Ukrainian National University